GEO AI –
Where AI Meets GIS
Derck Vonck is the Practice Lead for Analysis and AI and Liezel Botha is a Geospatial Technology Lead at Esri South Africa. In this interview, they share their insights on artificial intelligence (AI), machine learning and deep learning and their application in the South African context. They also look at how these techniques can work with geographic information systems (GIS) to unlock the strategic value of location data.
What exactly does Geo AI involve?
Geo AI involves using artificial intelligence techniques such as machine learning and deep learning on geospatial (location) data to unlock the value held in its spatial (where) and attribute (what, why) components.
AI is broadly defined as the use of computers to mimic human cognitive behaviour. It can involve tasks such as reasoning about facts, understanding language, robotics, classifying objects in images or recognising patterns in data or predicting outcomes based on past observations.
A subset of AI is machine learning where algorithms are trained on labelled data or find patterns in unlabelled data. The algorithms are then used to predict labels for data in unknown cases. An example of classification is when fruit is classified, based on its texture and weight, as either apples or oranges. This is a very simple example, but the same technique can be used for more complex cases such as classifying fraudulent credit cards transactions or the classification of features on aerial imagery such as swimming pools on properties.
An example of prediction would be to predict the revenue from a store based on features such as locality, size, demographics, time since its last refurbishment, etc. Using a technique such as random forest regression trees, it is possible to identify influential features that would determine a store’s revenue. Bringing in the “Geo” angle makes it possible to create features such as “population statistics around a store within a five minute drive time” using spatial analysis.
A new machine learning technique called deep learning neural networks has become very popular in the last five years. It has led to the creation of tools that exceed human cognition in the fields of image classification, object detection in images and translation between different languages. What sets deep learning apart from the machine learning techniques we’ve mentioned is that it creates its own features as part of the training. The depth of the neural network makes it possible to carry out classification, object detection or prediction procedures faster and more accurately than the average human could. Deep learning has been applied with great success to land classification, doing in a few hours what would otherwise have taken months of human labour.
How is Geo AI being used in South Africa?
One of the main uses of Geo AI in South Africa is analysing imagery for change detection. Geo AI makes rapid change detection analysis possible which in turn aids effective and timely decision making. Applications include the identification of backyard structures, rooftop solar panels, and instances of land encroachment on electrical servitudes. Change detection also plays an important role in the disaster management of communities affected by fires and flooding. Commercial applications of Geo AI include the analysis of sales and telematics data, site selection for new businesses, and the verification of fire or flood insurance claims.
How is Geo AI impacting GIS?
Geo AI is going to make the work of GIS professionals much easier by automating repetitive tasks such as data capture. Previously clients couldn’t access the full strategic value hidden in their data – processing limitations and time were just some of the constraints. With Geo AI, they can now use all their data, instead of just one or two variables. Results are also obtained so much faster. Geo AI will push GIS professionals beyond map and dashboard creation into analysis territory. Having good analytical skills will become increasingly important as will knowledge of scripting languages such as Python or R.
Are your clients making use of Geo AI?
Previously clients used imagery mainly for backdrop purposes, but now they want to know about change detection and classification of imagery. Looking for patterns in data with clustering tools has become so much easier and clients are appreciative of the value in being able to do this. The early adopters of Geo AI in South Africa are retailers, insurers, and mining companies. They are benefiting from enhanced operational efficiency in the current tight market conditions. We foresee more clients making use of the Geo AI tools on our platforms as they become more aware of the strategic value lying in their data. Geo AI used in conjunction with spatial analysis can help keep companies ahead of the competition.
How has Geo AI been incorporated into Esri platforms?
Clients are able to make use of Geo AI by using the new and improved change detection, clustering, and predictive modelling tools available on our platforms. Clients can simply run Esri’s out-of-the-box tools on their data and it will start revealing patterns.
How does Geo AI help with processing big data?
Many companies have so much data that they don’t know what to do with it. A retail organisation can have 300 shops around South Africa, and each of these shops will have 200 variables. Using clustering techniques, Geo AI can help retail companies to group their data together, so that patterns can become more obvious. Analysis of these patterns often leads to the identification of problems and possible solutions. For example, identifying where product is going missing during delivery rounds is something that can be addressed using clustering techniques and telematics data.
How did your first attempt at Geo AI go?
Our first Geo AI experience involved capturing pedestrian crossing data. We showed the computer a few examples of pedestrian crossings on aerial imagery and provided it with some rules. The computer then went ahead and identified pedestrian crossings on the imagery. A lot of time was saved by not having to pan and zoom in on all the imagery to find the crossings ourselves. We then verified the identifications only to discover that the answers were only around 75% accurate. After some investigating we realised our mistake; we hadn’t filtered the data according to the road network. The machine had done its job but had mistakenly identified white cars and empty parking spaces in parking lots as pedestrian crossings. This taught us the importance of filtering data to avoid false flagging, ensuring that the model does not overfit the data, and most critical of all, verifying the results.
What challenges do you foresee with Geo AI?
Very powerful techniques and software are available for creating prediction and classification models. Users should however be careful of bias in the data and do extensive cross validation on the models to ensure their accuracy. It is also quite easy to use these models to propagate existing biases in our understanding of the world. It’s also essential to understand that the models will only have value if they can be used to make changes in the real world. Esri’s platform makes it possible to easily get these powerful models into the hands of the end users.
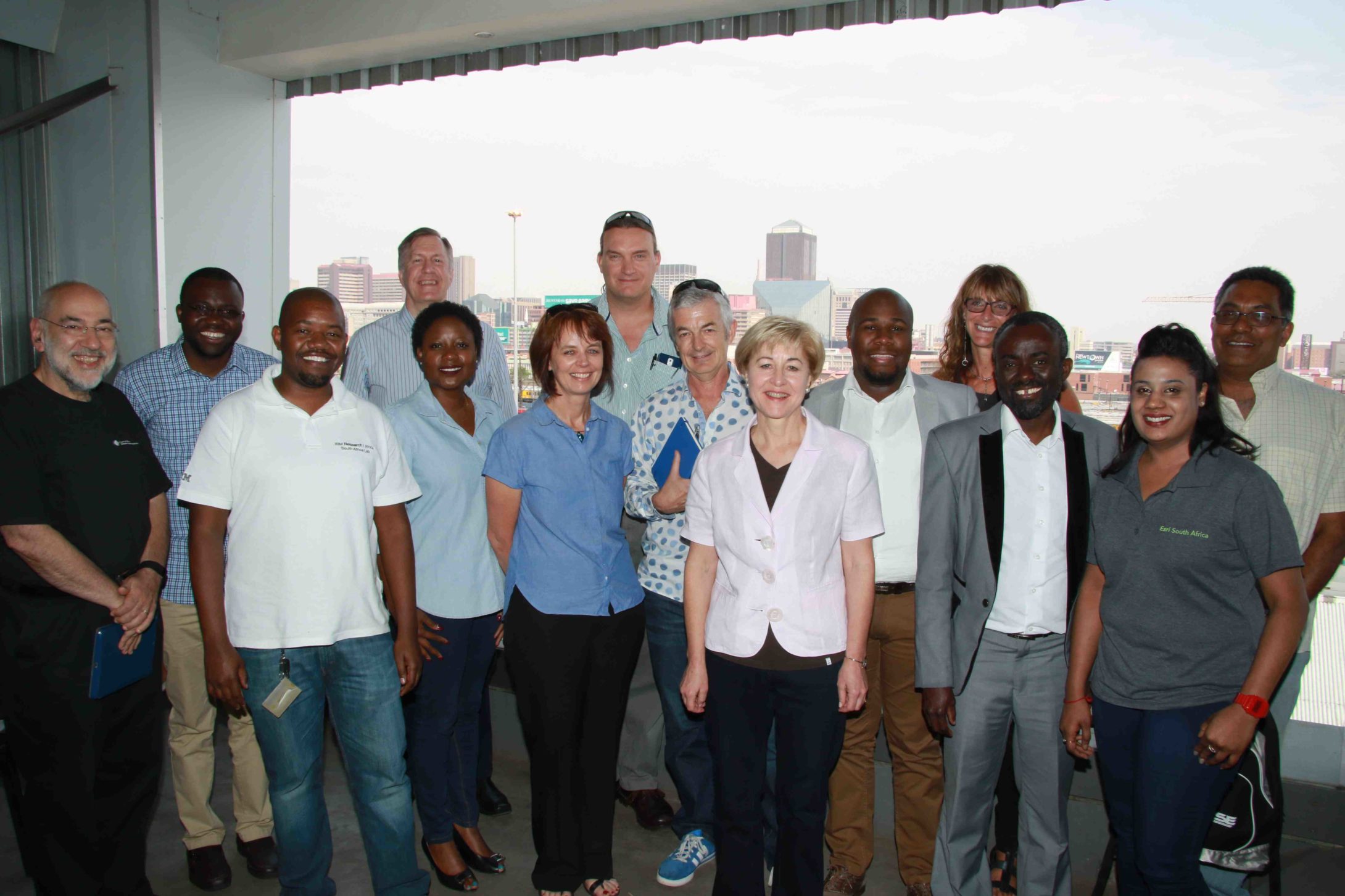